WebinarWatch on demand
Content-based Identifiers for Iterative Forecasts: A Proposal
Speaker
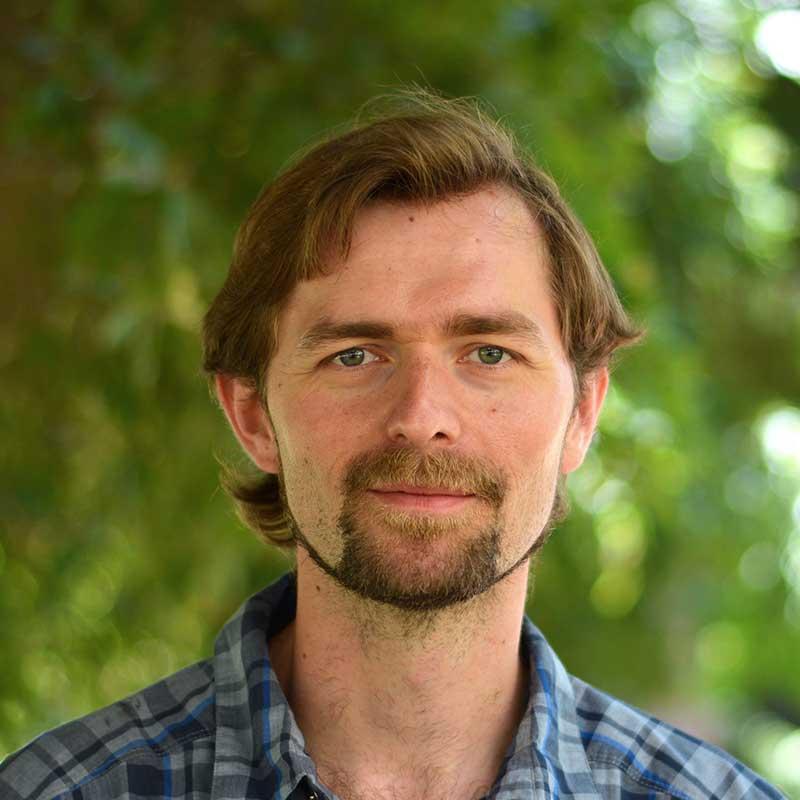
Carl Boettiger
University of California Berkeley
I work on problems in ecological forecasting and decision making under uncertainty, with applications for global change, conservation and natural resource management. I am particularly interested in how we can predict or manage ecological systems that may experience regime shifts: sudden and dramatic changes that challenge both our models and available data. The rapid expansion in both computational power and the available ecological and environmental data enables and requires new mathematical, statistical and computational approaches to these questions. Ecology has much to learn about what are and are not useful from advances in informatics & computer science, just as it has from statistics and mathematics. Traditional approaches to ecological modeling and resource management such as stochastic dynamic systems, Bayesian inference, and optimal control theory must be adapted both to take advantage of all available data while also dealing with its imperfections. My approach blends ecological theory with the synthesis of heterogeneous data and the development of software – a combination now recognized as data science.
Iterative forecasts pose particular challenges for archival data storage and retrieval. Content-based identifiers provide a convenient way to consistently identify input and outputs and associated scripts and in this webinar I will illustrate an example iterative forecasting workflow, including some newly developed R packages. Read more
Iterative forecasts pose particular challenges for archival data storage and retrieval. In an iterative forecast, data about the past and present must be downloaded and fed into an algorithm that will output a forecast data product. Previous forecasts must also be scored against the realized values in the latest observations. Content-based identifiers provide a convenient way to consistently identify input and outputs and associated scripts. These identifiers are:
- location agnostic – they don’t depend on a URL or other location-based authority (like DOI)
- reproducible – the same data file always has the same identifier
- frictionless – cheap and easy to generate with widely available software, no authentication or network connection
- sticky – the identifier cannot become unstuck or separated from the content
- compatible – most existing infrastructure, including DataONE, can quite readily use these identifiers. In this webinar I will illustrate an example iterative forecasting workflow. In the process, I will highlight some newly developed R packages for making this easier.
Watch previously recorded video